Professor Zoran Nikoloski from University of Potsdam is leading the CAPITALISE modelling work. He explains how the Bioinformatics work and modelling can help to extract value from the large heterogeneous datasets to aid understanding of the science and guide research efforts towards developing better crops more effectively.
Innovative plant breeding strategies are needed to address the dual problems of food security for a growing population and designing crops better able to mitigate the negative effects of a changing climate. Exploiting natural variability for yield and related crop performance traits provides a means to address this challenge. This approach entails first gathering of large, heterogeneous data for crop genetic resources to characterize their variability at the genomic, molecular, physiological, and performance level. Mathematical modelling approaches are in turn developed and applied to analyse the resulting data and to plan the next steps of crop improvement.
Mathematical modelling approaches for crop improvement can be categorized into: (1) statistical, (2) mechanistic, and (3) hybrid. This categorization reflects the type of problems addressed. For instance, statistical approaches aim to identify and model relationships between measured features and traits solely based on the measured data. Common representatives include the now classical machine learning approaches as well as modern deep learning techniques to solve regression and classification problems. In contrast, mechanistic approaches, as the name indicates, use the established knowledge of molecular mechanisms to identify the key determinants of studied traits. Mechanistic approaches can be classified based on the type of processes modelled (e.g. steady state vs. dynamic, stochastic vs. deterministic) and depend on system parameters that are either obtained from literature or are inferred based on the gathered data. What is common to both approaches is that they aim to predict yield-related traits for unseen individuals and/or environments that can guide the development of crop improvement strategies.
Crop improvement strategies rooted in statistical modelling rely on availability of genomic data and data about studied traits to first identify genetic basis of the studied traits. For instance, mapping approaches (e.g. genome-wide association) can pinpoint loci statistically associated with a given trait. As a result, they can propel the discovery of genetic and molecular mechanisms underlying the trait. In contrast, genomic and phenomic prediction approaches neglect the identification of loci controlling a trait and instead aim to identify individuals with desired traits based on purely data-driven models. In the context of CAPITALISE, we make use of both of these strategies to determine loci underlying photosynthetic efficiency as well as genotypes with improved performance with respect to photosynthesis-related traits. Since photosynthetic efficiency affects and is determined by multiple other traits, we also aim to develop statistical approach to predict multiple traits. As a result, we make novel use of heterogeneous data available from past projects and gathered as part of CAPITALISE. The resulting approaches can be used in any setting that entails the prediction of multiple traits from genomics data.
Improvement of photosynthetic efficiency with mechanistic models allows us to identify the bottlenecks of the underlying molecular and signalling pathways and to design strategies to overcome these bottlenecks. For instance, kinetic models of the Calvin-Benson cycle – the collection of metabolic reactions underpinning photosynthesis – have been used to determine reactions whose rate increase is predicted to increase photosynthetic rate. In addition, and in contrast with statistical modelling approaches, mechanistic models can be used to simulate unseen environments. However, the discovery of bottlenecks and simulations of different environments with the help of mechanistic models of cellular pathways requires knowledge about the parameters that denote key enzyme kinetic properties (e.g. turnover number) and can be used to describe the rate of the modelled biochemical reactions. To this end, data about the concentration of the modelled molecular components is needed to obtain crop- and individual-specific estimates of enzyme parameters. Nevertheless, the parameter values used in the existing models of photosynthesis are measured from in vitro assays that may differ in orders of magnitude compared to those from in vivo estimates. In the context of CAPITALISE, we aim to develop crop-specific models of photosynthesis, parameterized based on data gathered from our experiments. Such an approach, applied with data from multiple crop individuals, will allow us to also survey the variability in parameter values and identify those that distinguish individuals with low and high photosynthetic efficiency. Yet, while this approach makes excellent use of the collected molecular and physiological data, it does not explore the potential of linking genomic data with enzymatic parameters for informed selection of better performing individuals, as done in the case of statistical models, above.
Hybrid modelling approaches rely on combining statistical and mechanistic modelling techniques to make full use of the big, heterogeneous data gathered from experiments surveying natural variability. In addition, hybrid models have the potential to simulate both unseen individuals and environments – essential for selection of individuals with traits tailored for future climate conditions. In CAPITALISE, we pursue a recently introduced hybrid modelling approach, called network genomic selection, that combines genomic prediction with mechanistic models of metabolism with the aim of improving the accuracy of growth prediction.
We expect that the development of modelling approaches for crop improvement in the next 5-10 years will include:
-
- identification of master control loci for multiple traits and prioritizing of loci for experimental validation by developing network-based approaches, further propelling the application of genome-wide association;
- integration of epistatic and environmental effects in genomic prediction models, improving the accuracy and widening the applicability of genomic and phenomic prediction approaches;
- mechanistic models at the level of individual and micro-environment, aligning the modelling developments with precision agriculture;
- strengthening the hybrid integration of machine / deep learning models with mechanistic models of cellular processes (beyond metabolism) to predict yield-related traits.
For instance, the latter can include genomic prediction of enzyme kinetic properties that can be integrated with large-scale models of crop metabolism to improve predictions of molecular traits (e.g. protein allocation) and growth, at the cost of requiring more data for model training.
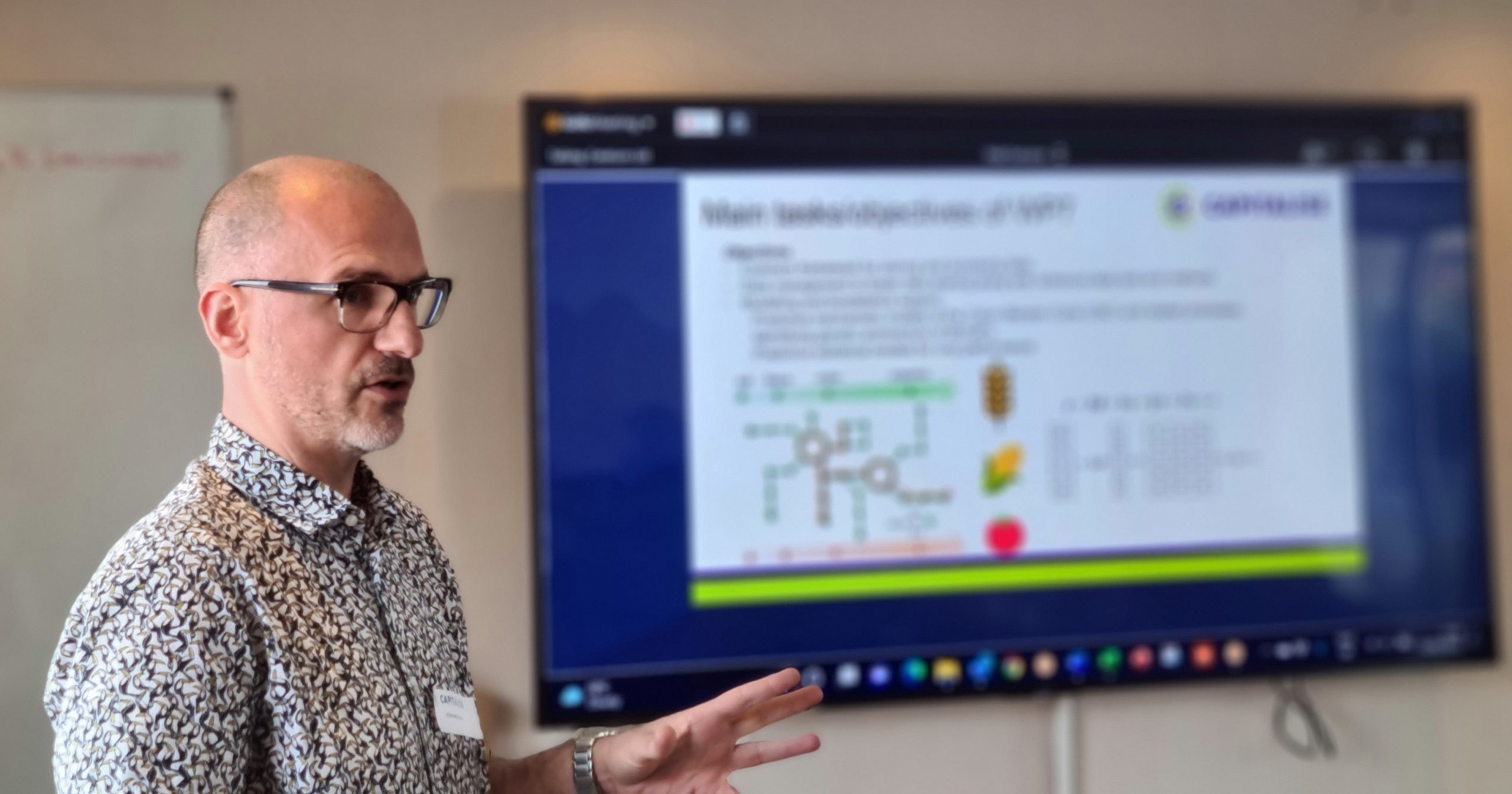